Brain Tumour Detection and Classffication Using CNN
Keywords:
Segmentation, Medical image, CNN, Machine learning, Classification, Barin tumourAbstract
The goal of this study is to find brain tumours and give better care to those who have them. Tumours are abnormal growths of cells in the brain, and cancer is the word for a type of malignant tumour. Most of the time, CT or MRI scans are used to find areas of cancer in the brain. Positron Emission Tomography, Cerebral Arteriogram, Lumbar Puncture, and Molecular testing are also used to find brain tumours. In this study, the dataset has 253 Brain MRI images in two folders called "yes" and "no". The "yes" folder has 155 Brain MRI images of tumours, while the "no" folder has 98 Brain MRI non-tumour images. For classification and segmentation of preprocessing augmented data set we use novel CNN architecture in this paper we have achieved a Training accuracy is 98.75% and the validation accuracy is 86.45%. The novel CNN architecture could be used as a useful decision-support tool for radiologists in medical diagnostics.
Downloads
References
David N. Louis, Arie Perry, et al., “The 2016 World Health Organization Classification of Tumors of the Central Nervous System: a summary”, Acta Neuropathol, Springer may 2016
Salander, P., Bergenheim, A. T., Hamberg, K., & Henriksson, R. (1999). Pathways from symptoms to medical care: a descriptive study of symptom development and obstacles to early diagnosis in brain tumour patients. Family Practice, 16(2), 143-148.
McKinney, P. A. (2004). Brain tumours: incidence, survival, and aetiology. Journal of Neurology, Neurosurgery & Psychiatry, 75(suppl 2), ii12-ii17.
Heimans, J. J., & Taphoorn, M. J. (2002). Impact of brain tumour treatment on quality of life. Journal of neurology, 249(8), 955-960.
Suresh, M., Sinha, A., & Aneesh, R. P. (2019). Real-Time Hand Gesture Recognition Using Deep Learning. International Journal of Innovations and Implementations in Engineering, 1.
Gurbina, M., Lascu, M., & Lascu, D. (2019, July). Tumor detection and classification of MRI brain image using different wavelet transforms and support vector machines. In 2019 42nd International Conference on Telecommunications and Signal Processing (TSP) (pp. 505-508). IEEE.
Somasundaram S and Gobinath R, “Early Brain Tumour Prediction using an Enhancement Feature Extraction Technique and Deep Neural Networks”, International Journal of Innovative Technology and Exploring Engineering (IJITEE), ISSN: 2278- 3075, Volume-8, Issue-10S, August 2019.
Damodharan, S., & Raghavan, D. (2015). Combining tissue segmentation and neural network for brain tumor detection. International Arab Journal of Information Technology (IAJIT), 12(1).
Hemanth, G., Janardhan, M., & Sujihelen, L. (2019, April). Design and implementing brain tumor detection using machine learning approach. In 2019 3rd International Conference on Trends in Electronics and Informatics (ICOEI) (pp. 1289-1294). IEEE.
Mathew, A. R., & Anto, P. B. (2017, July). Tumor detection and classification of MRI brain image using wavelet transform and SVM. In 2017 International conference on signal processing and communication (ICSPC) (pp. 75-78). IEEE.
Mathew, A. R., & Anto, P. B. (2017, July). Tumor detection and classification of MRI brain image using wavelet transform and SVM. In 2017 International conference on signal processing and communication (ICSPC) (pp. 75-78). IEEE.
Chakrabarty, N. (2019). Brain mri images for brain tumor detection. Kaggle.
Poornachandra, S., & Naveena, C. (2017, March). Pre-processing of mr images for efficient quantitative image analysis using deep learning techniques. In 2017 International Conference on Recent Advances in Electronics and Communication Technology (ICRAECT) (pp. 191-195). IEEE.
Al Okashi, O. M., Mohammed, F. M., & Aljaaf, A. J. (2019, October). An Ensemble Learning Approach for Automatic Brain Hemorrhage Detection from MRIs. In 2019 12th International Conference on Developments in eSystems Engineering (DeSE) (pp. 929-932). IEEE.
Soumya, R. S., Neethu, S., Niju, T. S., Renjini, A., & Aneesh, R. P. (2016, July). Advanced earlier melanoma detection algorithm using colour correlogram. In 2016 International Conference on Communication Systems and Networks (ComNet) (pp. 190-194). IEEE.
J. A. Akhila, C, Markose, et al. "Feature extraction and classification of Dementia with neural network," 2017 International Conference on Intelligent Computing, Instrumentation and Control Technologies (ICICICT), Kerala, India, 2017, pp. 1446-1450
Sinha, A., & Aneesh, R. P. (2019). Real time facial emotion recognition using deep learning. International Journal of Innovations and Implementations in Engineering, 1.
Nandpuru, H. B., Salankar, S. S., & Bora, V. R. (2014, March). MRI brain cancer classification using support vector machine. In 2014 IEEE Students' Conference on Electrical, Electronics and Computer Science (pp. 1-6). IEEE.
El-Dahshan, E. S. A., Hosny, T., & Salem, A. B. M. (2010). Hybrid intelligent techniques for MRI brain images classification. Digital signal processing, 20(2), 433-441.
W. H. Ibrahim, A. A. A. Osman, and Y. I. Mohamed, ‘‘MRI brain image classification using neural networks,’’ in Proc. Int. Conf. Comput., Electr. Electron. Eng. (ICCEEE), Aug. 2013, pp. 253–258.
Rajini, N. H., & Bhavani, R. (2011, June). Classification of MRI brain images using k-nearest neighbor and artificial neural network. In 2011 International conference on recent trends in information technology (ICRTIT) (pp. 563-568). IEEE.
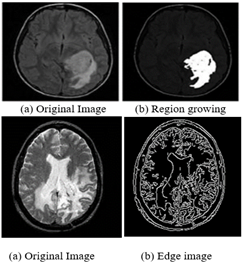
Downloads
Published
How to Cite
Issue
Section
License
Copyright (c) 2022 Neetu Nagar, Nishi Pandey, Abhishek Agwekar

This work is licensed under a Creative Commons Attribution 4.0 International License.
Copyright on any article in the International Journal of Engineering and Applied Physics is retained by the author(s) under the Creative Commons license, which permits unrestricted use, distribution, and reproduction provided the original work is properly cited.
License agreement
Authors grant IJEAP a license to publish the article and identify IJEAP as the original publisher.
Authors also grant any third party the right to use, distribute and reproduce the article in any medium, provided the original work is properly cited.