A Survey on Various Brain MR Image Segmentation Techniques
Keywords:
Segmentation, Medical image, Speckle noise, Fuzzy Clustering Technique, Brain MR ImageAbstract
Prior to medical image analysis, segmentation is an essential step in the preprocessing process. Partitioning an image into distinct regions based on characteristics like texture, color, and intensity is its primary goal. Numerous applications include tumor and coronary border recognition, surgical planning, tumor volume measurement, blood cell classification and heart image extraction from cardiac cine angiograms are all made possible by this technique. Many segmentation methods have been proposed recently for medical images. Thresholding, region-based, edge-based, clustering-based and fuzzy based methods are the most important segmentation processes in medical image analysis. A variety of image segmentation methods have been developed by researchers for efficient analysis. An overview of widely used image segmentation methods, along with their benefits and drawbacks, is provided in this paper.
Downloads
References
M. Xess, S. A. Agnes, “Survey on Clustering Based Color Image Segmentation and Novel Approaches To Fcm Algorithm”, IJRET: International Journal of Research in Engineering and Technology, pp. 346-349. 1
Kavitha, P., & Saraswathi, P. V. (2020). Segmentation for Content Based Satellite Image Retrieval using Fuzzy Clustering. International Journal of Advanced Science and Technology, 29. 2
Manisha, P., Jayadevan, R., & Sheeba, V. S. (2020, April). Content-based image retrieval through semantic image segmentation. In AIP Conference Proceedings (Vol. 2222, No. 1, p. 030008). AIP Publishing LLC. 3
Mistry, Y. D. (2020). Textural and color descriptor fusion for efficient content-based image retrieval algorithm. Iran Journal of Computer Science, 3(3), 169-183. 4
Sultana, F., Sufian, A., & Dutta, P. (2020). Evolution of image segmentation using deep convolutional neural network: a survey. Knowledge-Based Systems, 201, 106062. 5
Ali, H. M., Kaiser, M. S., & Mahmud, M. (2019, December). Application of convolutional neural network in segmenting brain regions from mri data. In International Conference on Brain Informatics (pp. 136-146). Springer, Cham. 6
Xu, Y., Wang, Y., Yuan, J., Cheng, Q., Wang, X., & Carson, P. L. (2019). Medical breast ultrasound image segmentation by machine learning. Ultrasonics, 91, 1-9. 7
Bi, L., Kim, J., Ahn, E., Kumar, A., Feng, D., & Fulham, M. (2019). Step-wise integration of deep class-specific learning for dermoscopic image segmentation. Pattern recognition, 85, 78-89. 8
Remeseiro, B., & Bolon-Canedo, V. (2019). A review of feature selection methods in medical applications. Computers in biology and medicine, 112, 103375. 9
D Kaur and Yadwinder Kaur “Various Image Segmentation techniques: A Review, in International Journal of Computer Science and Mobile Computing (IJCSMC), vol. 3, pp 809- 814, 2014. 10
Manoharan, S. (2020). Performance analysis of clustering-based image segmentation techniques. Journal of Innovative Image Processing (JIIP), 2(01), 14-24. 11
Wang, Z., Wang, E., & Zhu, Y. (2020). Image segmentation evaluation: a survey of methods. Artificial Intelligence Review, 53(8), 5637-5674. 12
S Inderpal and K Dinesh, “A Review on Different Image segmentation Techniques” IJAR, vol. 4, 2014. 13
Gonzalez Rafel C, Richard E Woods, “Digital Image Processing” Pearson Education, 3rd Edition, 2007. 14
Pare, S., Kumar, A., Singh, G. K., & Bajaj, V. (2020). Image segmentation using multilevel thresholding: a research review. Iranian Journal of Science and Technology, Transactions of Electrical Engineering, 44(1), 1-29. 15
Xu, L. Wang, S. Feng, and Y. Qu, "Threshold-based level set method of image segmentation on Intelligent Networks and Intelligent Systems (ICINIS), pp. 703-706, 2010. 16
Sharif M, Tanvir U, Munir EU, Khan MA, Yasmin M (2018) Brain tumor segmentation and classification by improved binomial thresholding and multi-features selection. J Ambient Intell Human Comput:1–20 17
F. C. Monteiro and A. Campilho, "Watershed framework to region-based image segmentation," in Proc. International Conference on Pattern Recognition, ICPR 19th, pp. 1-4, 2008. 18
R. Patil and K. Jondhale, "Edge based technique to estimate number of clusters in k- means color image segmentation," in Proc. 3rd IEEE International Conference on Computer Science and Information Technology (ICCSIT), pp. 117-121, 2010. 19
Fabijanska, "Variance filter for edge detection and edge-based image segmentation," in Proc. International Conference on Perspective Technologies and Methods in MEMS Design (MEMSTECH), pp. 151-154, 2011. 20
M. J. Islam, S. Basalamah, M. Ahmadi, and M. A. S. hmed, "Capsule image segmentation in pharmaceutical applications using edge-based techniques," IEEE International Conference on Electro/Information Technology (EIT), pp. 1- 5, 2011. 21
M. SHARIF, M. RAZA, and S. MOHSIN, "Face recognition using edge information and DCT, "Sindh Univ. Res. Jour.(Sci. Ser.), vol. 43, no. 2, pp. 209-214,2011. 22
Gupta, D., & Anand, R. S. (2017). A hybrid edge-based segmentation approach for ultrasound medical images. Biomedical Signal Processing and Control, 31, 116-126. 23
Baziyad, M., Rabie, T., & Kamel, I. (2020, April). Achieving stronger compaction for dct-based steganography: A region-growing approach. In World Conference on Information Systems and Technologies (pp. 251-261). Springer, Cham. 24
S. M. M. Sharif, M. J. Jamal, M. Y. Javed, and M. Raza, "Face recognition for disguised variations using gabor feature extraction, "Australian Journal of Basic and Applied Sciences, vol. 5, pp. 1648-1656, 2011. 25
M. Sharif, S. Mohsin, M. Y. Javed, and M. A. Ali, "Single image face recognition using Laplacian of Gaussian and discrete cosine transforms," Int. Arab J. Inf. Technol., vol. 9, pp. 562-570, 2012. 26
Joo L, Jung SC, Lee H, Park SY, Kim M, Park JE et al (2021) Stability of MRI radiomic features according to various imaging parameters in fast scanned T2-FLAIR for acute ischemic stroke patients. Sci Rep 11:1–11 27
Park JG, Lee C (2009) Skull stripping based on region growing for magnetic resonance brain images. Neuroimage 47:1394–1407 28
Menze BH, Jakab A, Bauer S, Kalpathy-Cramer J, Farahani K, Kirby J et al (2014) The multimodal brain tumor image segmentation benchmark (BRATS). IEEE Trans Med Imaging 34:1993–2024 29
Manoharan, S. (2020). Performance analysis of clustering-based image segmentation techniques. Journal of Innovative Image Processing (JIIP), 2(01), 14-24. 30
Hassan, M. R., Ema, R. R., & Islam, T. (2017). Color image segmentation using automated K-means clustering with RGB and HSV color spaces. Global Journal of Computer Science and Technology. 31
Basar, S., Ali, M., Ochoa-Ruiz, G., Zareei, M., Waheed, A., & Adnan, A. (2020). Unsupervised color image segmentation: A case of RGB histogram-based K-means clustering initialization. Plos one, 15(10), e0240015. 32
Celebi M E, Kingravi H A and Vela P A,” A comparative study of efficient initialization methods for the k-means clustering algorithm”. Expert Systems with Applications”, 2013, 40(1) pp. 200-210. 33
Reddy, M., Makara, V., & Satish, R. U. V. N. (2017). Divisive Hierarchical Clustering with K-means and Agglomerative Hierarchical Clustering. Int J of Comp Science Trands and Tech (IJCST), 5(5), 5-11. 34
Tokuda, E. K., Comin, C. H., & Costa, L. D. F. (2020). Revisiting Agglomerative Clustering. arXiv preprint arXiv:2005.07995. 35
Ghufron, G., Surarso, B., & Gernowo, R. (2020). The Implementations of K-medoids Clustering for Higher Education Accreditation by Evaluation of Davies Bouldin Index Clustering. Jurnal Ilmiah KURSOR, 10(3). 36
Šostak, A., Uljane, I., & Eklund, P. (2020, June). Fuzzy Relational Mathematical Morphology: Erosion and Dilation. In International Conference on Information Processing and Management of Uncertainty in KnowledgeBased Systems (pp. 712-725). Springer, Cham. 37
Balado, J., Van Oosterom, P., Díaz-Vilariño, L., & Meijers, M. (2020). Mathematical morphology directly applied to point cloud data. ISPRS Journal of Photogrammetry and Remote Sensing, 168, 208-220. 38
Srinivasan, A., & Sadagopan, S. (2020). Rough fuzzy region based bounded support fuzzy C-means clustering for brain MR image segmentation. Journal of Ambient Intelligence and Humanized Computing, 1-14. 39
S. Kobashi and J. K. Udupa, "Fuzzy object model based fuzzy connectedness image segmentation of newborn brain MR images," in Proc. IEEE International Conference on Systems, Man, and Cybernetics (SMC), pp. 1422- 1427, 2012. 40
Nafisuddin Khan., & Arya, K. V. (2020). A new fuzzy rule-based pixel organization scheme for optimal edge detection and impulse noise removal. Multimedia Tools and Applications, 1-27. 41
M. R. Khokher, A. Ghafoor, and A. M. Siddiqui, "Image segmentation using fuzzy rule-based system and graph cuts," in Proc. 12th International Conference on Control Automation Robotics & Vision (ICARCV), pp. 1148- 1153, 2012. 42
M. Sharif, S. Mohsin, M. J. Jamal, and M. Raza, "Illumination normalization preprocessing for face recognition," in Proc. International Conference on Environmental Science and Information Application Technology (ESIAT), pp. 44-47, 2010. 43
Sarma, R., & Gupta, Y. K. (2021). A comparative study of new and existing segmentation techniques. In IOP Conference Series: Materials Science and Engineering (Vol. 1022, No. 1, p. 012027). IOP Publishing. 44
Agrawal, A. P., & Tyagi, N. (2020). REVIEW ON DIGITAL IMAGE SEGMENTATION TECHNIQUES. Journal of Critical Reviews, 7(3), 779-784. 45
Jeevitha, K., Iyswariya, A., RamKumar, V., Basha, S. M., & Kumar, V. P. (2020). A REVIEW ON VARIOUS SEGMENTATION TECHNIQUES IN IMAGE PROCESSSING. European Journal of Molecular & Clinical Medicine, 7(4), 1342-1348. 46
Abbasi S, Tajeripour F (2017) Detection of brain tumor in 3D MRI images using local binary patterns and histogram orientation gradient. Neurocomputing 219:526–535 48
Manoharan, S. (2020). Performance analysis of clustering-based image segmentation techniques. Journal of Innovative Image Processing (JIIP), 2(01), 14-24. 49
Hassan, M. R., Ema, R. R., & Islam, T. (2017). Color image segmentation using automated K-means clustering with RGB and HSV color spaces. Global Journal of Computer Science and Technology. 50
Basar, S., Ali, M., Ochoa-Ruiz, G., Zareei, M., Waheed, A., & Adnan, A. (2020). Unsupervised color image segmentation: A case of RGB histogram-based K-means clustering initialization. Plos one, 15(10), e0240015. 51
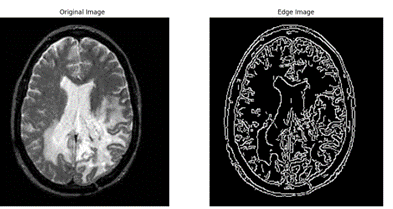
Downloads
Published
How to Cite
Issue
Section
License
Copyright (c) 2022 Neetu, Nishi Pande, Abhishek Agvekar

This work is licensed under a Creative Commons Attribution 4.0 International License.
Copyright on any article in the International Journal of Engineering and Applied Physics is retained by the author(s) under the Creative Commons license, which permits unrestricted use, distribution, and reproduction provided the original work is properly cited.
License agreement
Authors grant IJEAP a license to publish the article and identify IJEAP as the original publisher.
Authors also grant any third party the right to use, distribute and reproduce the article in any medium, provided the original work is properly cited.