Fault Detection Analysis in Ball Bearings using Machine Learning Techniques
Keywords:
Misalignment, Fault Detection, Bearing, Machine LearningAbstract
The Bearing element is very essential component of any rotating equipment. Any defect in the bearings lead to instable performance of the machinery. To avoid such malfunction and breakdown of the machinery equipment due to misalignment is review critically in this research paper and various machine learning techniques to tackle the issue is highlighted. This review article finds the basis for developing an effective system in order to reduce the breakdown of machinery or equipment. Conventional Machine Learning methods, like Artificial neural network, Decision Tree, Random Forest, Support Vector Machines(SVM) have been applied to detecting categorizing fault, while the application of Deep Learning methods has ignited great interest in the industry.
Downloads
References
Junning Li, Jiafan Xue, Ka Han, Qian Wang and Wuge Chen, “Experimental Analysis on Skid Damage of Roller Bearing with the Time-Varying Slip and Temperature Distribution” Appl. Sci, 18 December 2019.
Her-Terng Yau, Ying-Che Kuo, Chieh-Li Chen, Yu-Chung Li “Ball bearing test-rig research and fault diagnosis investigation” IET Science, Measurement & Technology, 2016.
Avinash V. Patil, Dr. Bimlesh Kumar, “Study Of Fault Diagnosis On Inner Surface Of Outer Race Of Roller Bearing Using Acoustic Emission”, International Research Journal of Engineering and Technology (IRJET), June -2017.
Nouby M. Ghazaly, G. T. Abd el- Jaber, Nadica Stojanovic, “Study Various Defects of Ball Bearings through Different Vibration Techniques”, American Journal of Mechanical Engineering, 2019.
Sham Kulkarni, Anand Bewoor, “Vibration based condition assessment of ball bearing with distributed defects”, Journal of Measurements in Engineering, JUNE 2016.
Sakshi Kokil, M. M. Shah, S. Y. Gajjal, S. D. Kokil, “Detection of Fault in Rolling Element Bearing Using Condition Monitoring by Experimental Approach”, International Journal of Engineering Research & Technology August – 2014.
Iulian LUPEA, Mihaiela LUPEA,“Fault Detection On A Rotating Test Rig Based On Vibration Analysis And Machine Learning” PROCEEDINGS OF THE ROMANIAN ACADEMY,2022.
T. Narendiranath, Babu T. Manvel Raj, T. Lakshmanan “A Review on Application of Dynamic Parameters of Journal Bearing for Vibration and Condition Monitoring” Journal of Mechanics, August 2015
Eugenio Brusa, Cristiana Delprete, Lorenzo Giorio, Luigi Gianpio Di Maggio and Vittorio Zanella “Design of an Innovative Test Rig for Industrial Bearing Monitoring with Self-Balancing Layout” Machines 2022.
Chao Fu1, Kuan Lu1, Yongfeng Yang, Zhongliang Xie, Anbo Ming1, “Nonlinear Vibrations of an Uncertain Dual Rotor Rolling Bearings System with Coupling Misalignment”, Journal of Nonlinear Mathematical Physics,2022.
A. M. Umbrajkaar, A. Krishnamoorthy,1 and R. B. Dhumale2 “Vibration Analysis of Shaft Misalignment Using Machine Learning Approach under Variable Load Conditions” Shock and Vibration,2020.
Chandrabhanu Malla, Isham Panigrahi1, “Review of Condition Monitoring of Rolling Element Bearing Using Vibration Analysis and Other Technique, Journal of Vibration Engineering & Technologies, 15 May 2019.
Youfu Tang, Feng Lin,and Qian Zou, “Complexity Analysis of Time-Frequency Features for VibrationSignals of Rolling Bearings Based on Local Frequency” , Shock and Vibration,2019.
Nader Sawalhi, Suri Ganeriwala, Máté Tóth, “Parallel misalignment modeling and coupling bending stiffness measurement of a rotor-bearing system”, Applied Acoustics,2017.
Vivek Parmar, V Huzur Saran, SP Harsha, “Effect of dynamic misalignment on the vibration response, trajectory followed, and defect-depth achieved by the rolling-elements in a double-row spherical rolling-element bearing”, Mechanism and Machine Theory, 20 April 2021.
Shen Zhang, Shibo Zhang, Bingnan Wang, Thomas G. Habetler, “Deep Learning Algorithms for Bearing Fault Diagnostics – A Comprehensive Review”, 6 Feb 2020.
Duy-Tang Hoang, Hee-Jun Kang, “A Survey on Deep Learning based Bearing Fault Diagnosis”, Neurocomputing, 10 June 2018.
Jiedi Sun, Changhong Yan, and Jiangtao Wen, “Intelligent Bearing Fault Diagnosis Method Combining Compressed Data Acquisition and Deep Learning”, IEEE TRANSACTIONS ON INSTRUMENTATION AND MEASUREMENT, September 4, 2017.
Rafia Nishat Toma, Alexander E. Prosvirin and Jong-Myon Kim, “Bearing Fault Diagnosis of Induction Motors Using a Genetic Algorithm and Machine Learning Classifiers”, Sensors,28 March 2020.
Miguel Delgado, Giansalvo Cirrincione, Antonio Garcia Espinosa, Juan Antonio Ortega, Humberto Henao “Bearing Faults Detection by a Novel Condition Monitoring Scheme based on Statistical-Time Features and Neural Networks”, August 26, 2012
Sailendu Biswal, “Design and Development of a Wind Turbine Test Rig for Condition Monitoring Studies” International Conference on Industrial Instrumentation and Control, 2015.
Y.S. Lee, C.W. Lee, “Modelling and vibration analysis of misaligned rotor-ball bearing systems”, Journal of Sound and Vibration, 1999.
Zijian Qiao, Zhengrong Pan, “SVD principle analysis and fault diagnosis for bearings based on the correlation coefficient”, Measurement Science and Technology, 2015.
P K Kankar, Satish C Sharma, S P Harsha, “Rolling element bearing fault diagnosis using autocorrelation and continuous wavelet transform”, Journal of Vibration and Control, 2011.
Mao Kunli, Wu Yunxin, “Fault Diagnosis of Rolling Element Bearing Based on Vibration Frequency Analysis”, Third International Conference on Measuring Technology and Mechatronics Automation, 2011.
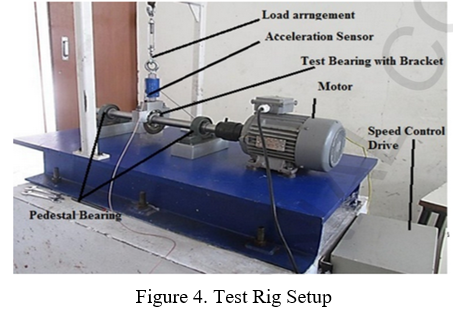
Downloads
Published
How to Cite
Issue
Section
License
Copyright (c) 2023 Shubham Junghare

This work is licensed under a Creative Commons Attribution 4.0 International License.
Copyright on any article in the International Journal of Engineering and Applied Physics is retained by the author(s) under the Creative Commons license, which permits unrestricted use, distribution, and reproduction provided the original work is properly cited.
License agreement
Authors grant IJEAP a license to publish the article and identify IJEAP as the original publisher.
Authors also grant any third party the right to use, distribute and reproduce the article in any medium, provided the original work is properly cited.
Similar Articles
- Victor Antwi, Dynamic Force Analysis on Blades of Centrifugal Pumps using Computational Fluids Dynamics Simulations , International Journal of Engineering and Applied Physics: Vol. 3 No. 2: May 2023
You may also start an advanced similarity search for this article.