The parameter update of Lithium-ion battery by the RSL algorithm for the SOC estimation under extended kalman filter (EKF-RLS)
Keywords:
Battery parameter estimation, Parameter identification, Battery management system, State-of-charge, Extended kalman filter, Recursive least square method, Lithium-ion batteryAbstract
The lithium-ion battery is the key power source of an electric vehicle. The cornerstone of safe transportation vehicles is reliable real-time state of charge (SOC) information. Since batteries are the primary form of energy storage in electric vehicles (EVs) and the smart grid, estimation of the state of charge is a critical need for batteries. The SOC estimate approach is considered to be precise and simple to apply for such applications. In this paper, After studying a battery model with an appropriate resistor-capacitor (RC) circuit, A lookup table derived from experimental studies describes the nonlinear connection between the Open Circuit Voltage Voc and the the state of charge. However, if temperature or SOC varies, the equivalent circuit model's characteristics will vary, decreasing the accuracy of SOC calculation. The recursive least squares (RLS) and nonlinear Extended Kalman filters are used in this research to offer a charge estimate technique with online parameter identification to handle this problem. RLS dynamically updates the Thevenin model's parameters. In order to improve the precision of SOC prediction under charge and discharge settings, we presented a regression least-squares-extended Kalman filter (RLS-EKF) estimation approach in this study. The objective of this research is to ensure the updating of the battery parameters and to evaluate the influence of this improvement on the convergence of the state of charge towards the real value. The simulation results suggest that the RLS EKF estimation technique, which is based on precise modeling, may greatly increase SOC estimation accuracy.
Downloads
References
S. Han, B. Zhang, X. Sun, S. Han, and M. Höök, “China’s Energy Transition in the Power and Transport Sectors from a Substitution Perspective,” Energies, vol. 10, no. 5, Art. no. 5, May 2017, doi: 10.3390/en10050600.
M. M. Hoque, M. A. Hannan, A. Mohamed, and A. Ayob, “Battery charge equalization controller in electric vehicle applications: A review,” Renew. Sustain. Energy Rev., vol. 75, no. C, pp. 1363–1385, 2017.
“A New BMS Architecture Based on Cell Redundancy | IEEE Journals & Magazine | IEEE Xplore.” https://ieeexplore.ieee.org/document/5645678 (accessed Mar. 06, 2023).
L. Lu, X. Han, J. Li, J. Hua, and M. Ouyang, “A review on the key issues for lithium-ion battery management in electric vehicles,” J. Power Sources, vol. 226, pp. 272–288, Mar. 2013, doi: 10.1016/j.jpowsour.2012.10.060.
G. Plett, “Extended Kalman filtering for battery management systems of LiPB-based HEV battery packsPart 1. Background,” J. Power Sources, Jun. 2004, doi: 10.1016/S0378-7753(04)00359-3.
R. Xiong, H. He, F. Sun, and K. Zhao, “Evaluation on State of Charge Estimation of Batteries With Adaptive Extended Kalman Filter by Experiment Approach,” IEEE Trans. Veh. Technol., vol. 62, no. 1, pp. 108–117, Jan. 2013, doi: 10.1109/TVT.2012.2222684.
F. Zhang, G. Liu, L. Fang, and H. Wang, “Estimation of Battery State of Charge With ?nfty Observer: Applied to a Robot for Inspecting Power Transmission Lines,” IEEE Trans. Ind. Electron., vol. 59, no. 2, pp. 1086–1095, Feb. 2012, doi: 10.1109/TIE.2011.2159691.
A. Alfi, M. Charkhgard, and M. Haddad Zarif, “Hybrid state of charge estimation for lithium-ion batteries: design and implementation,” IET Power Electron., vol. 7, no. 11, pp. 2758–2764, 2014, doi: 10.1049/iet-pel.2013.0746.
H. He, X. Zhang, R. Xiong, Y. Xu, and H. Guo, “Online model-based estimation of state-of-charge and open-circuit voltage of lithium-ion batteries in electric vehicles,” Energy, vol. 39, no. 1, pp. 310–318, Mar. 2012, doi: 10.1016/j.energy.2012.01.009.
W. Waag, C. Fleischer, and D. U. Sauer, “Critical review of the methods for monitoring of lithium-ion batteries in electric and hybrid vehicles,” J. Power Sources, vol. 258, pp. 321–339, Jul. 2014, doi: 10.1016/j.jpowsour.2014.02.064.
H. Rahimi-Eichi, U. Ojha, F. Baronti, and M.-Y. Chow, “Battery Management System: An Overview of Its Application in the Smart Grid and Electric Vehicles,” IEEE Ind. Electron. Mag., vol. 7, no. 2, pp. 4–16, Jun. 2013, doi: 10.1109/MIE.2013.2250351.
“Energies | Free Full-Text | Online Parameter Identification and State of Charge Estimation of Lithium-Ion Batteries Based on Forgetting Factor Recursive Least Squares and Nonlinear Kalman Filter.” https://www.mdpi.com/1996-1073/11/1/3 (accessed Jan. 09, 2023).
“Adaptive parameter identification and State-of-Charge estimation of lithium-ion batteries.” https://ieeexplore.ieee.org/abstract/document/6389248/ (accessed Jan. 09, 2023).
L. Zhang, X. Hu, Z. Wang, F. Sun, and D. G. Dorrell, “A review of supercapacitor modeling, estimation, and applications: A control/management perspective,” Renew. Sustain. Energy Rev., vol. 81, pp. 1868–1878, Jan. 2018, doi: 10.1016/j.rser.2017.05.283.
“Energies | Free Full-Text | A New Method for State of Charge Estimation of Lithium-Ion Batteries Using Square Root Cubature Kalman Filter.” https://www.mdpi.com/1996-1073/11/1/209 (accessed Jan. 09, 2023).
S. Panchal, I. Dincer, M. Agelin-Chaab, M. Fowler, and R. Fraser, “Uneven temperature and voltage distributions due to rapid discharge rates and different boundary conditions for series-connected LiFePO4 batteries,” Int. Commun. Heat Mass Transf., vol. 81, pp. 210–217, Feb. 2017, doi: 10.1016/j.icheatmasstransfer.2016.12.026.
W.-Y. Chang, “The State of Charge Estimating Methods for Battery: A Review,” ISRN Appl. Math., vol. 2013, pp. 1–7, Jul. 2013, doi: 10.1155/2013/953792.
I. Baccouche, S. Jemmali, B. Manai, R. Chaibi, and N. E. Ben Amara, “Hardware implementation of an algorithm based on kalman filtrer for monitoring low capacity Li-ion batteries,” in 2016 7th International Renewable Energy Congress (IREC), Mar. 2016, pp. 1–6. doi: 10.1109/IREC.2016.7478930.
Z. Yu, R. Huai, and L. Xiao, “State-of-Charge Estimation for Lithium-Ion Batteries Using a Kalman Filter Based on Local Linearization,” Energies, vol. 8, no. 8, Art. no. 8, Aug. 2015, doi: 10.3390/en8087854.
W. Zhang, W. Shi, and Z. Ma, “Adaptive unscented Kalman filter based state of energy and power capability estimation approach for lithium-ion battery,” J. Power Sources, vol. 289, pp. 50–62, Sep. 2015, doi: 10.1016/j.jpowsour.2015.04.148.
I. Reid and H. Term, “1 Discrete-time Kalman ?lter”.
M. Partovibakhsh and G. Liu, “An Adaptive Unscented Kalman Filtering Approach for Online Estimation of Model Parameters and State-of-Charge of Lithium-Ion Batteries for Autonomous Mobile Robots,” IEEE Trans. Control Syst. Technol., vol. 23, no. 1, pp. 357–363, Jan. 2015, doi: 10.1109/TCST.2014.2317781.
A. Vahidi, A. Stefanopoulou, and H. Peng, “Recursive least squares with forgetting for online estimation of vehicle mass and road grade: theory and experiments,” Veh. Syst. Dyn., vol. 43, no. 1, pp. 31–55, Jan. 2005, doi: 10.1080/00423110412331290446.
K. J. Åström and B. Wittenmark, Adaptive Control: Second Edition. Courier Corporation, 2013.
H. Rahimi-Eichi, F. Baronti, and M.-Y. Chow, “Online Adaptive Parameter Identification and State-of-Charge Coestimation for Lithium-Polymer Battery Cells,” IEEE Trans. Ind. Electron., vol. 61, no. 4, pp. 2053–2061, Apr. 2014, doi: 10.1109/TIE.2013.2263774.
R. Xiong, F. Sun, X. Gong, and C. Gao, “A data-driven based adaptive state of charge estimator of lithium-ion polymer battery used in electric vehicles,” Appl. Energy, vol. 113, pp. 1421–1433, Jan. 2014, doi: 10.1016/j.apenergy.2013.09.006.
H. Harmoko et al., “Online Battery Parameter And Open Circuit Voltage (OCV) Estimation Using Recursive Least Square (RLS),” Techné J. Ilm. Elektrotek., vol. 15, no. 01, Art. no. 01, Apr. 2016, doi: 10.31358/techne.v15i01.141.
W. He, N. Williard, C. Chen, and M. Pecht, “State of charge estimation for electric vehicle batteries using unscented kalman filtering,” Microelectron. Reliab., vol. 53, no. 6, pp. 840–847, Jun. 2013, doi: 10.1016/j.microrel.2012.11.010.
Z. Zeng, J. Tian, D. Li, and Y. Tian, “An Online State of Charge Estimation Algorithm for Lithium-Ion Batteries Using an Improved Adaptive Cubature Kalman Filter,” Energies, vol. 11, no. 1, Art. no. 1, Jan. 2018, doi: 10.3390/en11010059.
he Hongwen, R. Xiong, H. Guo, and S. Li, “Comparison study on the battery models used for the energy management of batteries in electric vehicles,” Energy Convers. Manag., vol. 64, pp. 113–121, Dec. 2012, doi: 10.1016/j.enconman.2012.04.014.
Z. Wei, C. Zou, F. Leng, B. Soong, and K. Tseng, “Online Model Identification and State-of-Charge Estimate for Lithium-Ion Battery With a Recursive Total Least Squares-Based Observer,” IEEE Trans. Ind. Electron., vol. PP, pp. 1–10, Aug. 2017, doi: 10.1109/TIE.2017.2736480.
H. Harmoko et al., “Online Battery Parameter And Open Circuit Voltage (OCV) Estimation Using Recursive Least Square (RLS),” Techné J. Ilm. Elektrotek., vol. 15, no. 01, Art. no. 01, Apr. 2016, doi: 10.31358/techne.v15i01.141.
S. Herdjunanto, “Estimation of Open Circuit Voltage and electrical parameters of a battery based on signal processed by Recursive Least Square method using two separate forgetting factors,” 2016 6th Int. Annu. Eng. Semin. InAES, pp. 67–71, Aug. 2016, doi: 10.1109/INAES.2016.7821909.
M. Mara?, E. N. Ayvaz, and A. Özen, “A novel adaptive variable forgetting factor RLS algorithm,” in 2018 26th Signal Processing and Communications Applications Conference (SIU), May 2018, pp. 1–4. doi: 10.1109/SIU.2018.8404408.
H. Darong, K. Lanyan, M. Bo, Z. Ling, and S. Guoxi, “A New Incipient Fault Diagnosis Method Combining Improved RLS and LMD Algorithm for Rolling Bearings With Strong Background Noise,” IEEE Access, vol. 6, pp. 26001–26010, 2018, doi: 10.1109/ACCESS.2018.2829803.
S. Yang et al., “A parameter adaptive method for state of charge estimation of lithium-ion batteries with an improved extended Kalman filter,” Sci. Rep., vol. 11, p. 5805, Mar. 2021, doi: 10.1038/s41598-021-84729-1.
M. A. Hannan, M. S. H. Lipu, A. Hussain, and A. Mohamed, “A review of lithium-ion battery state of charge estimation and management system in electric vehicle applications: Challenges and recommendations,” Renew. Sustain. Energy Rev., vol. 78, pp. 834–854, Oct. 2017, doi: 10.1016/j.rser.2017.05.001.
P. Spagnol, S. Rossi, and S. M. Savaresi, “Kalman Filter SoC estimation for Li-Ion batteries,” 2011 IEEE Int. Conf. Control Appl. CCA, pp. 587–592, Sep. 2011, doi: 10.1109/CCA.2011.6044480.
D. Sun et al., “State of charge estimation for lithium-ion battery based on an Intelligent Adaptive Extended Kalman Filter with improved noise estimator,” Energy, vol. 214, p. 119025, Jan. 2021, doi: 10.1016/j.energy.2020.119025.
M.-K. Tran, A. DaCosta, A. Mevawalla, S. Panchal, and M. Fowler, “Comparative Study of Equivalent Circuit Models Performance in Four Common Lithium-Ion Batteries: LFP, NMC, LMO, NCA,” Batteries, vol. 7, no. 3, Art. no. 3, Sep. 2021, doi: 10.3390/batteries7030051.
Z. Cui, W. Hu, G. Zhang, Z. Zhang, and Z. Chen, “An extended Kalman filter based SOC estimation method for Li-ion battery,” Energy Rep., vol. 8, pp. 81–87, Aug. 2022, doi: 10.1016/j.egyr.2022.02.116.
H. Zhang, H. W. Mu, Y. Zhang, and J. Han, “Calculation and Characteristics Analysis of Lithium Ion Batteries’ Internal Resistance Using HPPC Test,” Adv. Mater. Res., vol. 926–930, pp. 915–918, 2014, doi: 10.4028/www.scientific.net/AMR.926-930.915.
V.-H. Duong, H. A. Bastawrous, K. Lim, K. W. See, P. Zhang, and S. X. Dou, “Online state of charge and model parameters estimation of the LiFePO4 battery in electric vehicles using multiple adaptive forgetting factors recursive least-squares,” J. Power Sources, vol. 296, pp. 215–224, Nov. 2015, doi: 10.1016/j.jpowsour.2015.07.041.
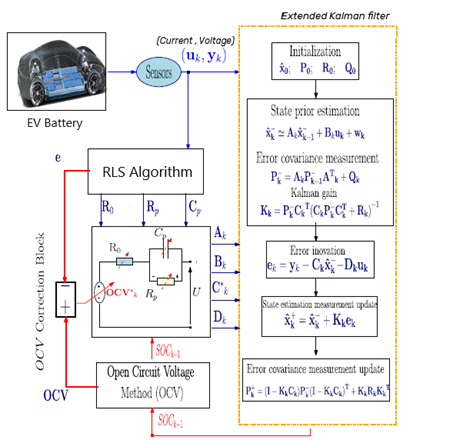
Downloads
Published
How to Cite
Issue
Section
ARK
License
Copyright (c) 2023 elmehdi Nasri, Tarik Jarou, Jawad Abdouni, Salma Benchikh, Sofia Elidrissi

This work is licensed under a Creative Commons Attribution 4.0 International License.
Copyright on any article in the International Journal of Engineering and Applied Physics is retained by the author(s) under the Creative Commons license, which permits unrestricted use, distribution, and reproduction provided the original work is properly cited.
License agreement
Authors grant IJEAP a license to publish the article and identify IJEAP as the original publisher.
Authors also grant any third party the right to use, distribute and reproduce the article in any medium, provided the original work is properly cited.
Similar Articles
- Subramaniyan Alagappan, Exploring the physics principles in cooking , International Journal of Engineering and Applied Physics: Vol. 3 No. 1: January 2023
You may also start an advanced similarity search for this article.